AI in drug discovery: why partnerships could hold the key to better biological data analysis
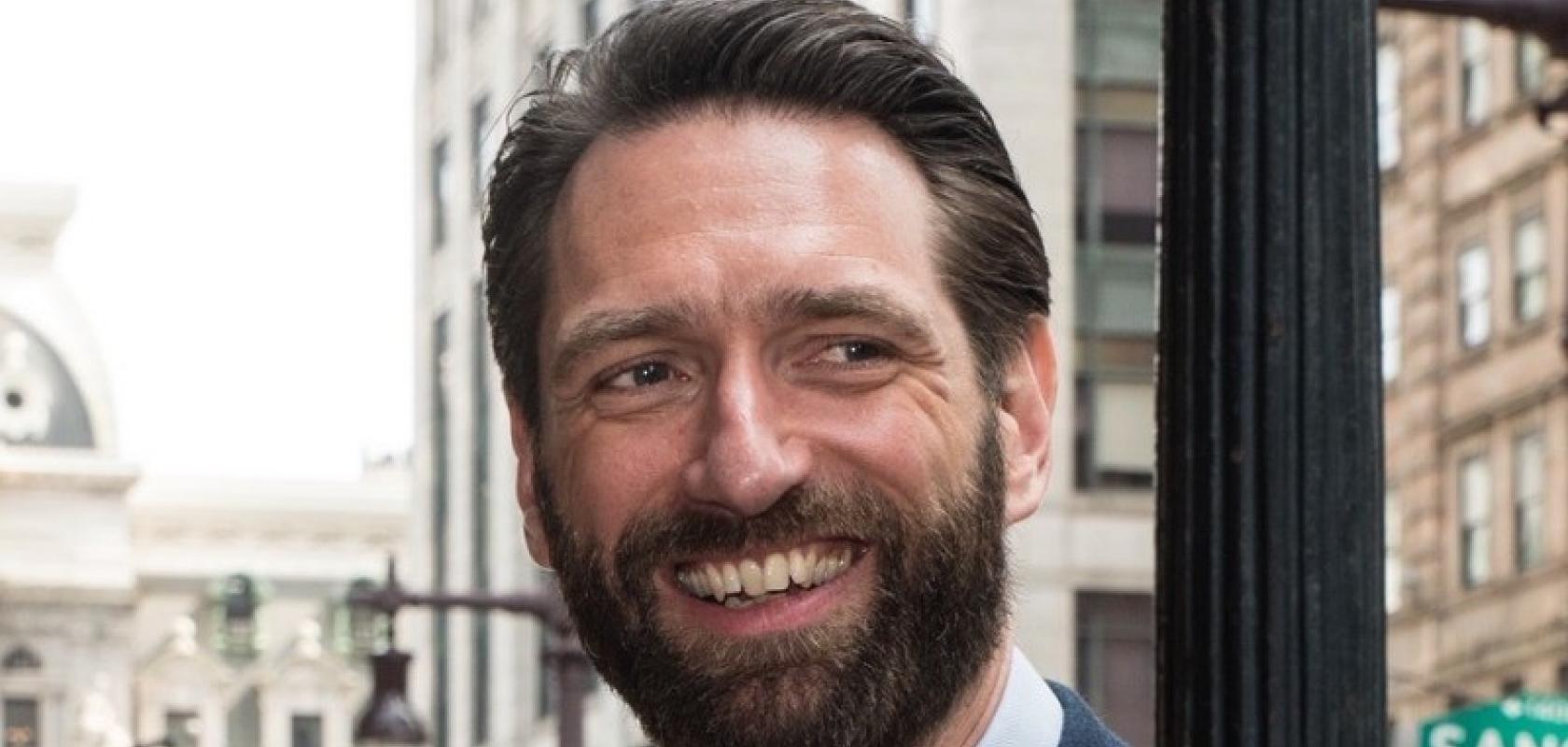
Dr Rob Grundy MBE, Director of Innovation Partnerships at Catalyst
Dr Rob Grundy MBE explains how collaborative approaches to AI implementation could help both large and small organisations overcome traditional drug discovery bottlenecks while avoiding costly technology investments
Register for FREE to unlock this article
Register with Scientific Computing World and gain unparalleled access to premium content and invaluable resources designed to keep you informed and ahead of the curve. By joining our community, you’ll enjoy FREE access to:
- Breaking news & updates: Keep up with the latest developments, market trends, and technological breakthroughs.
- Comprehensive articles & expert insights: Dive into detailed coverage of current trends, technologies, and innovations from leading experts in the field.
- Real-world case studies: Gain knowledge from real-world applications and success stories that highlight best practices and innovative solutions.
- Exclusive webinars & round tables: Be among the first to learn about and join upcoming webinars and round tables addressing key issues in scientific computing.
- In-depth reports & technical articles: Access insightful reports and whitepapers offering actionable information to support informed decision-making.
- Professional networking: Connect with a vibrant community of professionals, exchange ideas, and collaborate on projects.
Propel your career and projects forward with full articles and exclusive content. Sign up now to unlock Scientific Computing World’s premium access.
Sign up now - it’s fast, easy, and FREE!
Already registered? Log in here
You can see our full privacy policy here.
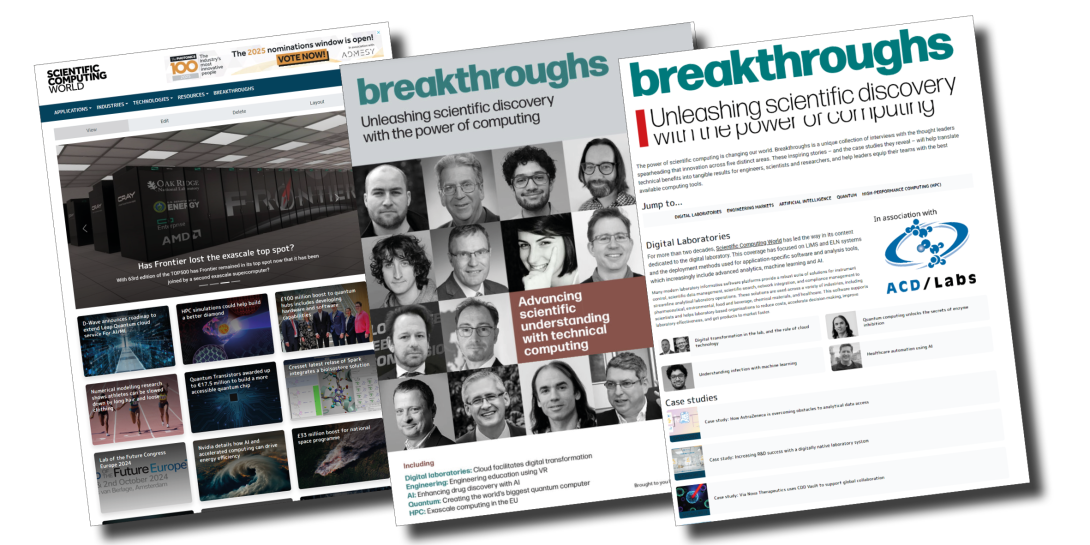